Data has become increasingly valuable across industries, especially with the extensive Internet use and smartphones. This data helps businesses understand users, develop strategies, and deliver services more efficiently.
In healthcare, data is particularly precious and highly targeted. When health systems, providers, and patients use these data, they can significantly improve care delivery and outcomes, especially when integrated with advanced analytics tools like artificial intelligence (AI).
Healthcare AI has garnered significant attention in recent years. Let’s discuss key use cases and the future of AI in healthcare.
Use of AI in Healthcare
Clinical Decision Support
Clinical decision support (CDS) systems are essential tools to enhance the quality of care and ensure patient safety. With the rapid advancements in technologies like artificial intelligence (AI) and machine learning (ML), these systems are revolutionizing how clinical decisions are made.
Initially, CDS tools were often standalone solutions that didn’t integrate well into clinical workflows. Nowadays, many CDS systems are embedded within electronic health records (EHRs), improving their deployment and maximizing their effectiveness at the patient’s bedside.
AI enhances this integration by leveraging information from both within the EHR and external data sources. AI tools can process vast amounts of data more efficiently than traditional methods, providing detailed insights that significantly impact clinical decision-making.
With AI’s advanced pattern recognition, CDS tools can incorporate risk stratification and predictive analytics. This allows clinicians to make more informed and personalized treatment recommendations, which are particularly valuable in managing chronic diseases.
Clinical Trials & Medical Research
Medical research is a cornerstone of the healthcare industry, driving the development of groundbreaking treatments and therapies. However, this research, especially clinical trials, demands significant financial, time, and resource investments.
AI tools can streamline data collection and management, break down data silos, optimize trial enrollment, and more, making them invaluable for medical research.
These technologies help accelerate clinical trials by improving the trial design, optimizing eligibility screening, and enhancing recruitment workflows. Additionally, AI models aid in advancing clinical trial data analysis, enabling researchers to process large datasets, detect patterns, predict results, and propose treatment strategies based on patient data.
Beyond the research realm, AI technologies also show promise in enhancing patient engagement.
Drug Discovery & Development
Drug discovery, development, and manufacturing have led to new treatments for many health conditions. Integrating AI and other technologies into these processes continues to revolutionize the pharmaceutical industry.
AI and other technologies can help address significant drug discovery and development barriers. AI and machine learning (ML) are transforming drug manufacturing by improving process optimization, predictive maintenance, and quality control. These technologies can identify data patterns humans might miss, thus enhancing efficiency.
In June 2023, research published in Science Advances showcased the potential of AI in drug discovery. The study revealed that a generative AI model could design novel molecules to block SARS-CoV-2, the virus causing COVID-19. The AI tool successfully identified drug-like molecules that bind with two COVID-19 protein targets responsible for virus transmission and spread.
The ability of AI models to analyze vast datasets is crucial not only for drug development but also for deriving insights from electronic health records (EHRs). This capability holds significant promise for advancing both fields.
Hospital Management
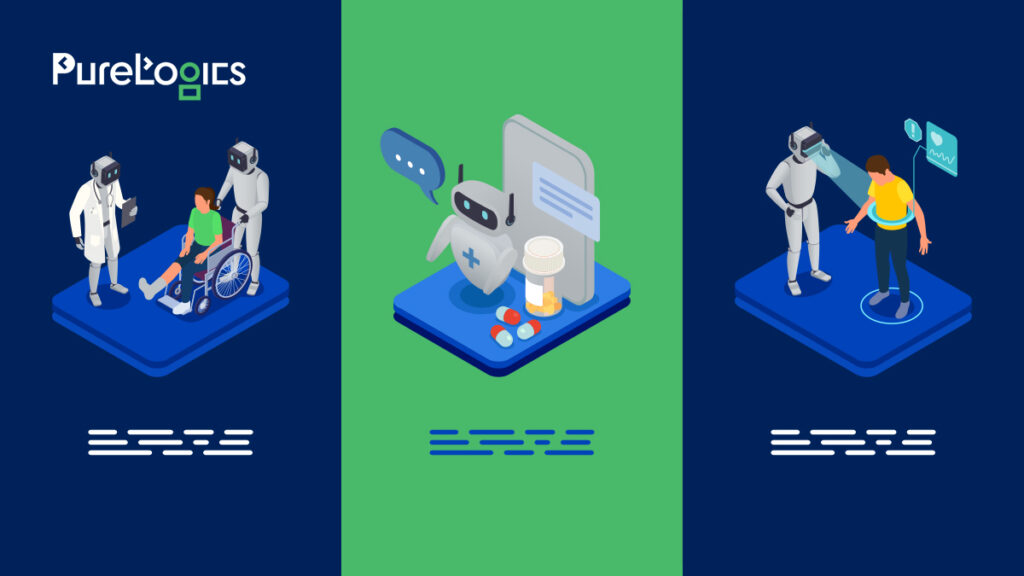
Managing health system operations and revenue cycles is crucial for delivering artificial intelligence in healthcare in the US. Optimizing workflows and monitoring capacity significantly impact a healthcare organization’s financial health and ability to provide high-quality care.
However, managing all necessary resources is a complex task, and health systems increasingly turn to data analytics solutions, such as AI, for assistance. Capacity management poses a significant challenge, especially with ongoing staffing shortages and the COVID-19 pandemic exacerbating existing hospital management issues like surgical scheduling.
Health systems must balance staffing limitations with surgeon preferences to address these challenges. Data analytics and AI can help navigate this complexity. Variations in surgeons’ operating room (OR) usage and scheduling preferences often result in inefficiencies, such as idle equipment, unused ORs, and surgeons struggling to secure OR block time.
AI and ML technologies have also advanced other aspects of AI in healthcare, such as medical imaging, enhancing overall efficiency and care quality.
Medical Imaging
Medical imaging plays a crucial role in diagnostics and pathology, but interpreting these images accurately requires significant clinical expertise and experience. Imaging analytics, often powered by AI, aims to address this challenge.
A study published in March 2024 by Johns Hopkins researchers in Communications Medicine demonstrated that a deep neural network-based automated detection tool could assist emergency room clinicians in diagnosing COVID-19 by analyzing lung ultrasound images.
The tool is designed to identify B-lines — bright, vertical image abnormalities that indicate inflammation in patients with pulmonary complications — to diagnose COVID-19 infection accurately. The success of this model suggests that a similar approach could be applied to other severe conditions, like heart failure, enabling efficient diagnosis at the point of care.
The researchers highlighted that such a capability would be highly beneficial when emergency department clinicians face high caseloads, such as during the early days of the COVID-19 pandemic. It could also be integrated into wearable technologies and other wireless devices for enhanced remote patient monitoring.
Patient Engagement
Effective communication is a crucial aspect of patient experience and engagement. Electronic Health Records (EHRs) facilitate this by allowing patients and providers to exchange messages anytime. However, the influx of messages can contribute to clinician burnout, as some queries are complex and time-consuming to address through EHR messages.
AI chatbots offer a potential solution by efficiently sorting patient needs and providing relevant resources. For instance, a health system might use a chatbot to filter patient phone calls handling simple requests like providing parking information to hospital visitors.
These AI tools can also address clinical needs using patient symptom data to recommend care.
AI-driven patient engagement can also include tools for conducting patient outreach based on clinical risk assessment data or translating health information for users in a patient portal. Often, these tools use predictive analytics to guide engagement efforts or generate valuable outputs.
Predictive Analysis
In recent years, predictive analytics has empowered artificial intelligence in healthcare providers to deliver more proactive patient care. Forecasting outcomes is crucial for developing important interventions and guiding clinical decision-making in the era of value-based care.
To effectively use predictive analytics, stakeholders must process vast amounts of high-quality data from various sources. Many predictive modeling tools incorporate AI to handle this data, offering numerous benefits and valuable use cases.
Predictive analytics enhances clinical decision support, population health management, and value-based care delivery, and its artificial intelligence in healthcare applications continues to expand. AI-based risk stratification plays a key role in these efforts by identifying patients at risk for adverse outcomes, thereby helping to prevent these outcomes and improve care quality.
In addition to predictive analytics, AI tools have significantly advanced the field of remote patient monitoring.
Future of AI in Healthcare
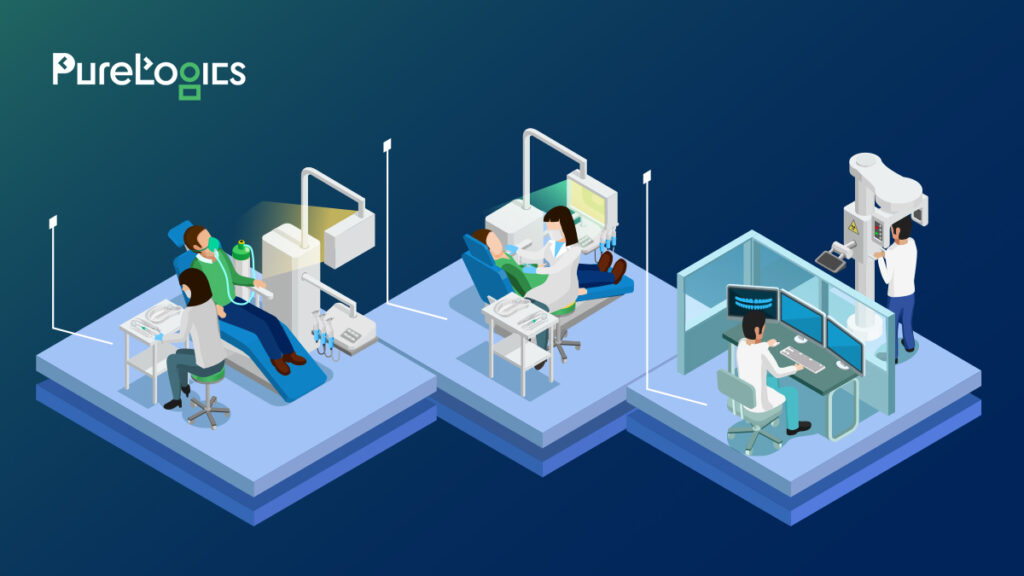
AI will play an imperative role in the future of healthcare. As the driving force behind precision medicine, AI, particularly machine learning, represents a much-needed advancement in care. While early attempts at providing diagnosis and treatment recommendations have been challenging, AI will eventually excel in this area.
With rapid advancements in AI for imaging analysis, machines will eventually examine most radiology and pathology images. Additionally, speech and text recognition are already being used for tasks like patient communication and capturing clinical notes, with their usage expected to grow.
The biggest challenge for AI in healthcare isn’t the capability of the technologies but ensuring their integration into daily clinical practice. For widespread adoption, AI systems must gain regulatory approval, integrate with EHR systems, standardize to ensure similar products function similarly, be taught to clinicians, be funded by public or private payers, and be continuously updated.
The development of chatbots is significantly advancing, helping patients by tracking their progress, sending tailored health notifications, and providing assistance through conversational AI. Some of these chatbots can even conduct entire virtual visits, assess symptoms, and direct patients to appropriate treatments or specialized appointments based on the information provided.
About PureLogics
Collaborating with a team that combines technological expertise and deep industry knowledge is crucial within AI in healthcare. PureLogics is a reliable technology partner with over two decades of industry experience. With a global team of professionals, PureLogics is well-equipped to provide the insights and solutions needed to drive success in healthcare. Give us a call today!
<p>The post How AI in Healthcare Takes the Industry Forward first appeared on PureLogics | Technology Services and Solutions.</p>